DEBLURMYIMAGE 1.51 FREE FREE DOWNLOAD
The referred work shows that even without a direct notion of a descriptor, a simple VGG with two inputs corresponding to 8 bits images is sufficient to learn a comparison function. To this purpose, the method takes as input a series of registered images and computes a weighted average of the Fourier coefficients of the images. On the other hand, the larger the region, the higher the probability of averaging out of context pixels information. Their algorithm returned a global score but involve a blurry reference image in the metric computation. Then, the probability that x i is blurrier than x j is calculated as: If a given Fourier coefficient in a frame is larger than its corresponding coefficients in the nearby frames, this indicates that it has been less attenuated by some motion blur. Based on this fact the multi-image deblurring task can be approached as an information aggregation problem where it is taken less blurred information of each frame to build an image that is sharper and less noisy than all the frames in the burst [ 5 ].
Uploader: | Goltiramar |
Date Added: | 28 October 2017 |
File Size: | 44.42 Mb |
Operating Systems: | Windows NT/2000/XP/2003/2003/7/8/10 MacOS 10/X |
Downloads: | 93236 |
Price: | Free* [*Free Regsitration Required] |
Then, the Point-Spread Function PSF h eeblurmyimage obtained by sampling the continuous trajectory on a regular pixel grid using subpixel linear interpolation, h: To work with variable burst lengths some authors performed a burst-size independent aggregation using a weighted average in the Fourier domain [ 5 ] and a recurrent network with temporal feature transference [ 1 ].
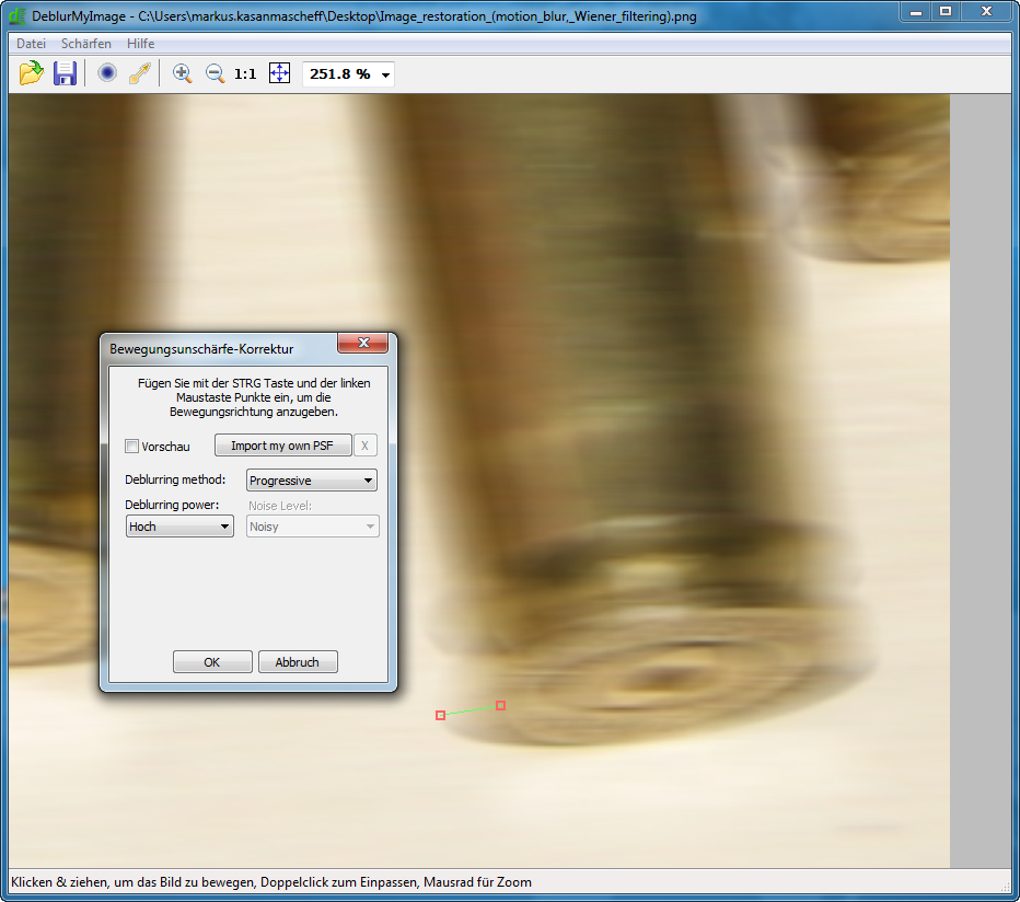
Despite the tree of these approaches, referred works do not consider that some images in the burst present severe blur degradation, misalignment even after registration or out-of-context frames that can lead to noisy reconstruction. Despite these metrics detect the amount of blur as expected in cases as seen in the Fig.
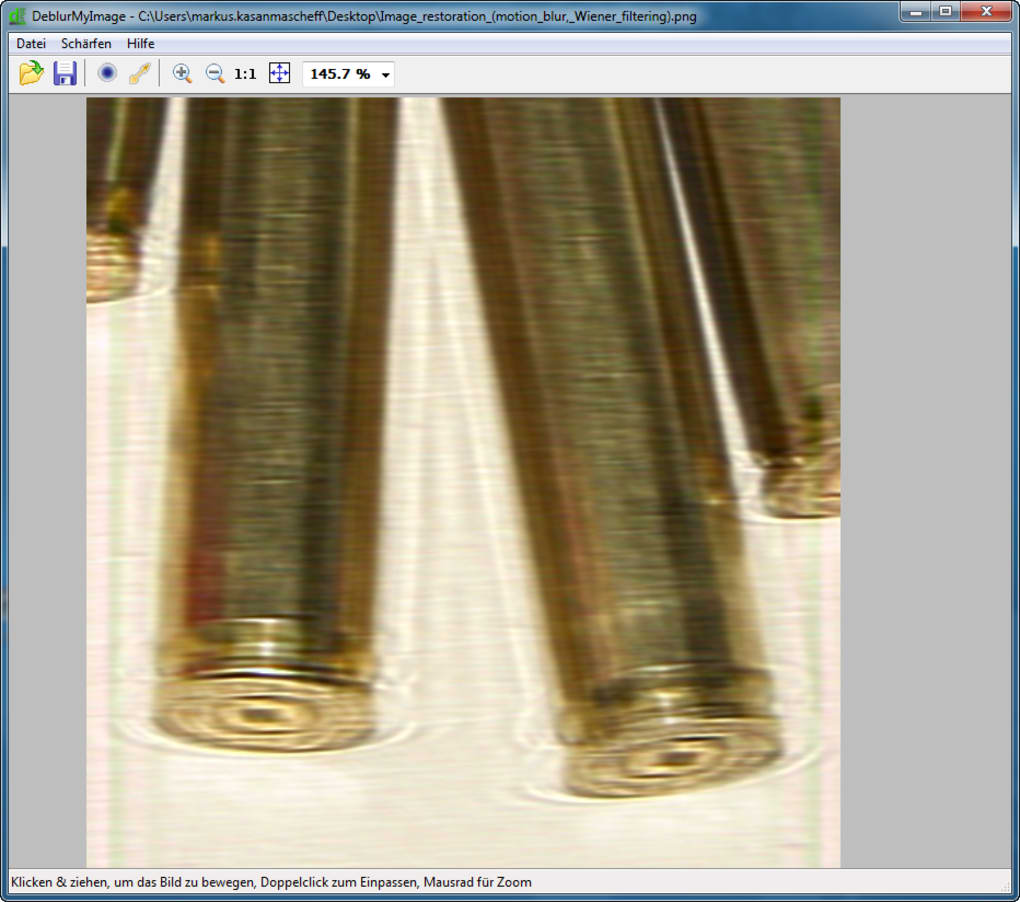
The method proposed in here deals with this problem introducing a new degradation recognition mechanism. It can be observed brightest reconstruction with fastMBD and FBA because all the frames within the burst in the reconstruction process were selected. We validate our findings on several synthetic and real datasets. The method is effective even if each image is blurred, as long as the blur is sufficiently time-variant to ensure that each Fourier coefficient has been left unaltered at least once in some neighboring frames [ 20 ].
Otherwise, the photons will be accumulated in neighboring pixels, generating a loss of sharpness blur. This incremental formulation of the FBA allow us to efficiently compute an iterative reconstruction with a degradation recognition mechanism.
Burst ranking for blind multi-image deblurring
Only images from deblurkyimage validation set of the MS COCO dataset were used in this phase for the synthetic burst generation. However, while in lucky methods the burst should contain at least one sharp image or region to obtain a proper reconstruction, the FBA fuses images within a burst by considering their frequency content without the need of sharp frames.
For such, the simplest approach is to compute a score r c i for a rfee image x i in the burst as: Quite the contrary, here an Incremental Fourier Burst Accumulation IFBA using only two images at each iteration along with an automatic image selection is proposed.
Our proposed degradation recognition mechanism is used to handle this situation. Also, the information aggregation is performed incrementally like in this work. Here, the reconstruction process can make use of additional data from neighboring images, but the problem is still challenging as each frame might encounter a different camera shake, and might not be aligned. However, is known that camera shake originated from hand tremor vibrations is essentially random [ 4 ].
As in [ 7 ]we test the deblurring method fixing the number of frames used during the aggregation. Image captured by hand-held camera devices usually contain motion blur, caused by a combination of camera shake and dynamic scene content [ 1 ]. This method allows using any classification 15.1 for sorting a set of images. We have found very expensive to create by hand the enormous amount of ground truth data required for the network.
For each image in the figure the respective values for the following quantities are presented: From a quick inspection of the figure it can be observed that from the aforementioned metrics a burst ranking cannot be obtained as in the manually sorted ground truth.
Burst ranking for blind multi-image deblurring
The sorting was made in 1. With this purpose, we recreate another common real-life situation where a burst is captured, and a moving object appears in the scene partially or entirely occluding the target object. The proposed incremental FBA, however, fuses all frames within the burst that do not cause a deterioration in the final image as expected. As can be seen in Table I our ranking function outperforms with high margin the current IQA metrics for recognizing the relative rank of blurred images.
It was demonstrated the superiority of our approach when compared to other similar methods in the wild. A comprehensive discussion about the B-T model and how to compute a global score given the pairwise comparison labels can be seen in [ 11 ] [ 24 ]. As mentioned above, we formulate the multi-image deblurring problem as a two steps process where it is performed a burst sorting as a local relative ranking task, and then an incremental aggregation method is applied.
Automatically selecting best frames within the burst to improve the base reconstruction is challenging because the amount of possible images fusions is equal to the power set cardinal. To measure the quantity of blur it is typical to use image quality metrics as shown in the Fig. Then, a metric is learned combining the information of each computed feature to meet the rank order given by the users.
In general, the more photons reach the surface, the better the quality of the final image, as the photonic noise is reduced. This network provides greater flexibility compared to the above models as it starts by processing the two images jointly. Experiments with the proposed algorithm have shown superior results when compared to other similar approaches, outperforming other methods described in the literature in previously described situations.
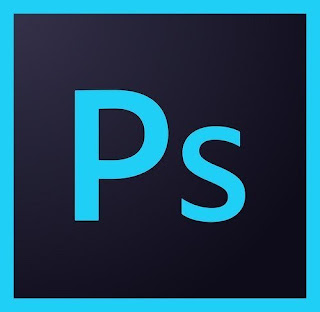
Comments
Post a Comment